
"I love Dr. Bryan Patrick Wood"
www.bpw1621.com VS www.gqak.com
2022-05-09 13:25:19
Home Vault Resume Extending Pandas Dr. Bryan Patrick Wood December 21, 2021 Filed under “DS/AI/ML” Open for extension, but closed for modification is a solid1 recommendation indeed.I love software that supports extension. I've had a particular fascination with extension mechanisms in softwarethroughout my career. I've also been lucky enough to work on several includingWriting plugins for a homegrown C++ data orchestration framework while at Raytheon SolipsysThis is before there were a lot of quality open-source data orchestration solutions available(e.g., Airflow, Nifi, etc.).Writing my own homegrown data orchestration framework from scratch in Java while at Focused SupportThis was pure joy: marrying my love for C++, Boost, and Python!Writing a framework to support Python scriptable components in a C++ application while at Stellar ScienceWriting a report generation framework in Python supporting pluggable section content using the pluggyMore on this in the future.and reportlab Python packages atmy current position with LeidosA predilection, mayhaps. Like a moth to the flame, when I discovered one of my most frequently used Python packagessupported extension mechanisms I was compelled to look into it.Who is this for?Although practicality beats purity2.Maybe you're not a software architecture wonk aficionado. That's fair: I'd speculate that's theexception rather than the rule for folks using pandas3. This is not a deep dive into how the extension mechanisms areimplemented. Interesting in their own right but out of the intended scope.The official documentation for extending pandas4 mentions four waysin which pandas can be extendedCustom accessorsCustom data typesSubclassing existing pandas data structuresThird-party plotting backendsThe focus here will be almost exclusively on #1: custom accessors. This is the one case I had a use for and think mostpandas users could benefit from knowing about.What are some reasons to continuing reading? Maybe you've noticed a few verbose pandasstatements that seem to recur often in your notebooks or scripts. Or maybe you're a teammate noticing the same mistakesor deviations from best practices during code review. Or maybe you're just a bit perverse and want to bend pandas toyour will. If this is you good reader, read on: what follows is practical information that can be applied to these ends.What is an Accessor?If you've used pandas for any amount of time you will already be familiar with them. pandas ships with a few builtinaccessors on the Series and DataFrame classesSeries.cat, Series.dt, Series.plot, Series.sparse, and Series.strDataFrame.plot and DataFrame.sparseI use Series.str5 all the time for manipulating string type columns in DataFrames. It providesvectorized string methods mirroring the Python standard library ones intelligently skipping over NAs. Series.plotand DataFrame.plot are another two that I use frequently whereas I have never had the occasion to use Series.sparseor DataFrame.sparse. The type of data you work with most often will dictate which will be most familiar so YMMV.Your Mileage May Vary(YMMV)Example usage is straight forwardprint(pd.Series(['bar', 'baz']).str.upper().str.replace('B', 'Z'))0 zAR1 zAZdtype: objectBoilerplatePandas supports registering custom accessors for its Index, Series, and DataFrame classes via decorators in thepandas.api.extensions module. These decorators expect a class which is passed the respective object as the onlynon-self argument to its __init__ method.Here is the src directory structureThe minimal boilerplate necessary for creating a pandas accessor for each supportedclass is shown in the figure below. If you only intend to write a DataFrame accessor the series.py and index.pymodules can be removed. The package naming and the accessor are both personalized with my initials. Since thiseffectively creates a namespace within the respectively classes it's first come, first served: sorry Bernard PiotrWojciechowski6. Viz., the pandas documentation for a geo DataFrame accessor that requires lat and loncolumns.4Including _validate methods is generally a good idea especially for special purpose accessors that only make sense touse on objects that meet enforceable preconditions. Since this is meant to be more of a grab bag of personalconveniences, validation will get pushed down to the individual accessor methods as appropriate obviating the need fortop level validation.With that in place we can verify everything is workingThe custom accessors do not have any useful methods or properties yet; however, at this point it's off to the races.What to Add?That's completely up to you: you now have your own pandas extension module. Pretty sweet! A good place to start islooking through your projects, scripts, notebooks, etc., that use pandas for patterns and repeated statements. Easytargets areAny functions that show up in frequently in pandas HoF calls (e.g., apply, map, applymap, aggregate,transform, etc.)Higher-order Function (HoF).pandas setup code can be pushed into the module __init__.pyProxies and aliasesStill stumped? I'll share some snippets for what I added to mine after creating theboilerplate to assist in your ideation process. I've elided details in the interest of brevity. For those details, thefull code can be found on GitHub and the package can be installed fromPyPI.Subject Matter Expert (SME).In my current position, the folks I'm working with are more likely to be SMEs in an area outside my own vice datascientists. As a result, I'm passed all sorts of unhygienic data and have amassed a variety of cleaning routines.Adding a common.py to collect these functions decoupled from their embedding in the accessorsPUNCTUATION_TO_SPACE_TRANSLATOR = str.maketrans(string.punctuation, ' ' * len(string.punctuation))DEFAULT_UNICODE_NORMALIZATION_FORM = 'NFKC'def clean_name(name: str): return '_'.join(name.lower().translate(PUNCTUATION_TO_SPACE_TRANSLATOR).split())def unicode_normalize(text: str, form: str = DEFAULT_UNICODE_NORMALIZATION_FORM): return unicodedata.normalize(form, text)Referencing it in series.py@register_series_accessor(ACCESSOR_NAME)class SeriesAccessor: @property def clean_name(self): series = self._series.copy() series.name = clean_name(series.name) return series def normalize(self, form: str = DEFAULT_UNICODE_NORMALIZATION_FORM): series = self._series.copy() return series.apply(functools.partial(unicode_normalize, form=form))And in dataframe.py@register_dataframe_accessor(ACCESSOR_NAME)class DataframeAccessor: @property def clean_names(self): df = self._df.copy() df.columns = [clean_name(column) for column in df.columns] return dfWe get our first set of useful custom accessor capabilities. clean_name and clean_namesWhile researching I found pyjanitor7 which has a more robust name cleaningcapability8. A ton of other useful stuff there: strongly encourage taking a look.should be pretty self-explanatory: lowercase, replace all special characters withspace, tokenize, and concatenate the tokens with underscores; normalize does Unicodenormalization9.Doing an expensive operation on a big DataFrame or Series? You're going to want an estimate of when it's likely toAn example of #2 above. There are certainly other (better?) ways to do this in interactive environments like ipython and jupyter startup scripts.be done. I've settled on tqdm10 for monitoring task progress in Python. It also conveniently has builtin supportfor pandas. However, this support needs to be initialized which can be easily dropped into the package __init__.pyas followsfrom tqdm.autonotebook import tqdmtqdm.pandas()sidetable, itself a pandas custom accessor, provides a few DataFrame summarizations:specifically, I use its freq method ... frequently. missingno provides visualizations for understanding the missinginformation in a DataFrame.Two packages I use frequently when analyzing data with pandas are the sidetable11 andmissingno12 packages.I usually want them around when I am using pandas. Baking them into the custom accessor is an easy way to do that aswell as provide some usage convenience.Here's the relevant codeclass _MissignoAdapter: def __init__(self, df: DataFrame): self._df = df def matrix(self, **kwargs): return missingno.matrix(self._df, **kwargs) def bar(self, **kwargs): return missingno.bar(self._df, **kwargs) def heatmap(self, **kwargs): return missingno.heatmap(self._df, **kwargs) def dendrogram(self, **kwargs): return missingno.dendrogram(self._df, **kwargs)@register_dataframe_accessor(ACCESSOR_NAME)class DataframeAccessor: @property def stb(self): return self._df.stb def freq(self, *args, **kwargs): return self._df.stb.freq(*args, **kwargs) @property def msno(self): return _MissignoAdapter(self._df)In the case of sidetable, this makes sure it is available after an import bpw_pde, provides an alias in the customaccessor to it, and pulls out the freq method to the top-level of the custom accessor. In the case of missingo, itsinterface needs adapting to work as a top-level msno propertyAll problems in computer science can be solved by another level of indirection, except for theproblem of too many layers of indirection.13on the custom accessor which is easily handled byintroducing a level of indirection through the adapter class.UsageThe package __init__.py imports all the individual accessor classes so registration of the custom accessorshappens on package import, i.e., import bpw_pde. Following that you can use the custom accessor just like the builtinones, e.g.,import pandas as pdimport matplotlib.pyplot as pltimport bpw_pdedf = pd.DataFrame(...)df = df.bpw.clean_namesdf.text = df.text.bpw.normalize()print(df.bpw.freq(['foo']))df.bpw.msno.matrix()plt.show()For additional usage, the package has tests which is always one of the first places to look when trying to figure outhow exactly to use some code.What Else?Plenty as you might imagine. As mentioned above there are four different ways to extend pandas. I've only touched onone in any detail here. A few final thoughts across all extension mechanisms follow.I'd strongly recommend checking out the pandas official documentation on the pandas ecosystem14.Most of the examples mentioned in the rest of this section can be found there as well as a treasure trove of unmentionedpandas goodies.Custom accessorsHopefully a well beaten horse at this point. I will site another blog post on this topic I came across and foundinteresting on thistopic for the unsated reader.I've already mentioned sidetable as a custom pandas accessor I use, and I've also created one, bpw_pde, you caninstall and use in your next project if you find it useful. pandas_path3 is another I'd recommendtaking a look at as a huge proponent and user of Python's bultin pathlib functionality.It would also be remiss not to mention an extension to pandas extension mechanism:pandas_flavor15 16. The primary additional functionality you get withpandas_flavor is the ability to register methods directly onto the underlying pandas type without an intermediateaccessor. Bad idea? I think it's probably safer to namespace your extensions vice effectivelymonkeypatching pandas coredata structures; however, I can also see the counterargument in exploratory data analysis environments.Context is everything.pyjanitor, mentioned above, uses pandas_flavor's direct method registration vice the builtin pandas customApplication Programming Interface (API).accessor extension API.Custom data typesThis is absolutely another extension mechanism I can see having a need for in the future that I did not know aboutbefore diving into this topic for the package and blog post. However, I didn't want to create a contrived one justfor the blog post because there are other good, useful open-source Python packages that do this. The documentatione.g., cyberpandas17 for custom data types related to IP Addresses.on this topic is pretty good and the ecosystem page has examples. I also recommend watchingthis dated but still relevant talk by core developer Will Ayd on the topic.Subclassing existing pandas data structuresThis is a much more time-consuming endeavor you'll need a good reason to pursue. To some degree, with custom accessors,this may be rarely needed in new code relegated mostly to the trash heap of legacy. Not a ton of good reasons come toBut, again, I can't think of a good reason why you'd need to do that either.mind but if you ever needed to override a pandas data structure method that might be one. A good example here thatI've used extensively is geopandas18.Third-party plotting backendsVery cool that this is supported but also incredibly niche. I can't imagine even most advanced users and developers ofpandas and related packages will ever need to use this. However, they may well benefit from it when a new plottingpackage developer can take advantage of it.SOLID ↩Zen of Python ↩pandas_path - Path style access for pandas ↩↩Extending pandas ↩↩pandas.Series.str ↩Bernard Piotr Wojciechowski ↩pyjanitor ↩pyjantior clean_names ↩Unicode normalization ↩tqdm documentation ↩Sidetable GitHub ↩Missingno GitHub ↩Fundamental theorem of software engineering ↩pandas ecosystem ↩pandas_flavor GitHub ↩pandas_flavor blog post ↩cyberpandas Read the Docs ↩GeoPandas Documentation ↩ Finalized at 11:35 AM. Tagged with data-science, Python, and programming. Click to read and post comments ? ORDAINED: The Python Project Template Dr. Bryan Patrick Wood October 24, 2021 Filed under “Programming” Creating a Python package from scratch is annoying. There is no standard library tooling to help. There is noauthoritative take on folder structure. So sling something into a single file script or Jupyter notebook tolanguish within Untitled7.ipynb to avoid the hassle. This did the job it needed to. Then it needs to be shared andreused ...All of this can be just enough friction to delay starting on a new idea. At least that has been the case for me.Let's even say a particularly motivated mood strikes. Putting together the project structure will be error-prone andrequire more effort searching the internet for arcane setup.cfg incantations than writing the actual code for theidea. Maybe that's all you have time to get done before it's off to other activities.Or worse: you don't even get that far.Not a great use of time. This should be the easy part!As a result of going through the process of spinning up a few new projects recently Idecided to take the time to better understand the Python packaging ecosystem and create a project boilerplatetemplate as an improvement over copying a directory tree and doing find and replace.Why?seems particularly popular.Is this reinventing the wheel? A valid question. Certainly somebody has already done this drudgery you say. And you'd beright. A quick web search will turn up pages of project templates. Same with githuband pypi. So the question remains. There are some good reasons in this case, some reasons I did notreach for something already available.First, I had been reading a book that went into detail on theis excellent and highly recommended (DISCLAIMER: I took this opportunity to look into affiliate marketing throughAmazon so the link above is one; if you care, it's easy enough to do an internet search on "serious python" and bypass).topic and felt like applying what I was learning: this is always a good reason.Second,this is the type of task a Python expert certainly should be comfortable executing; somewhat ironically, it'll alsooften be a task that is already taken care of at a company or on a project unless you're involved at a very earlystage.Third, I can't find the quote to do proper attribution unfortunately, but I recallreading something I'll paraphrase that resonated with meDon't use anything you can't take the time to learn well.Whether it's a 4,000+ line .vimrc file or a project template like this a time will almost certainly come when you needto change something. That's when the inevitable technical debt comes due and pay you will. My experience has been thatadding just what you need (and understand) and iterating over time is always a better strategy.Definitely not another case of ...Lastly, as I became more engrossed in the details of the endeavor, the point became tobe more opinionated especially with respect to dependent packages. I wanted something a coworker, colleague,collaborator, etc., could use immediately with my recommended dependencies for various different types of tasks. Turnsout this is straight forward to bake in.Familiarity in the sense that I had used someone else's cookiecutter template before.There are many possible approaches but the one I had already some familiarity with in the Python ecosystem wascookiecutter. From their messaging cookiecutter isA command-line utility that creates projects from cookiecutters (project templates), e.g. creating a Python packageproject from a Python package project template.Using a cookiecutter someone else has created is trivial as detailed in thedocumentationcookiecutter gh:bpw1621/ordainedis shorthand for accessing a github hostedcookiecutter template.cookiecutter https://github.com/bpw1621/ordainedorcookiecutter ordainedwhen the template has been pulled down already. Typically, you are greeted with a few questions to configure detailsabout the project template instantiation and then off to the races. For instance, the ordained cookiecutter templateprompts as followsI only needed to specify two options: the project name and description.Anyone other than me would have to enter all of their personal information but that can be handled with cookiecutter'ssupport for user config.The defaults attempt to be sane and minimize redundant data entry. At this point a fully functional Python package hasbeen created and the initial boilerplate version controlled in git. Since the options for specifying the type ofvirtual environment one wants at this point are a little complex that next step is left out of the automation (at leastat the moment, viz., below).Not sure the Python community has coalesced around cookiecutter as the solution, butit's at least a cut above copying an existing project and editing the various parts. Having used tools in otherAnd they have been improving, viz.,here.programming languages (e.g., Yeoman in Javascript) there is room for improvement. That said, oneof my favorite quotes isThe perfect is the enemy of the good.Le mieux est l'ennemi du bien.Voltaire, Philosophical DictionarySince the virtual environment creation is not automated a good default choice, after creating and activating theproject's virtual environment, is pip install -e .[base,dev,doc,test]. This will pull in those dependencies Itypically do not want to live without as a matter of quality of life (i.e., base), those integral fordevelopment (i.e., dev), those needed to generate documentation (i.e., doc), and those needed to test (i.e., test).Including any of the other requirements groups will depend on what the project is trying to accomplish.So What?A large part of the opinionated aspect resides in the specification of recommended project dependencies. This is accomplishedusing setuptools support for options.extras_require to provide groups of dependencies to pull in covering differenttopics. Those groups are specified in a requirements group dictionary as part of the cookiecutter JSON configuration.Here's the snippet from cookiecutter.jsonConfiguration keys in cookiecutter with two leading underscores stay part of the context butare suppressed from the initial configuration options provided to the user. This is unfortunately still an unreleasedfeature (as of cookiecutter 1.7.3) so using ordained requires installingcookiecutter from the HEAD of master (pre-release versionof 2.0.0 as of this writing).These topic based recommendations are very much a work in progress. It is largely informed, at the moment, by what Ihave been working on most recently and there are clearly large gaps. A hope is that as folks use this that it will bea wellspring of suggestions as to the Python packages I am not even aware that should be included as well as betteralternatives to those I have grown to rely on. I will put aside why I made these choices for a future post after therecommendations are a little more fully fleshed out. At any rate, if you have your own dependency packagerecommendations it is trivial to fork the project and change a single JSON object in the top-level cookiecutter.jsonwith them. The requirements group dictionary is used in a Jinja2 template to generate setup.cfgin the projectThere is some unseemly vanity in sharing some of these gory technical details just becauseI think they're clever. That said, it did take me sometime (viz., the - all over the place) to get it quiteright given I had never written a complex Jinja2 template like this before.This could have been jammed inline in the project template, but I think it is cleaner to leaveit here and less digging through the guts of the template to make additions and modifications.which creates the requirements groups lexicographically sorted with a special all group for the kitchen sink.Here are some other capability highlights provided directly out of the boxsrc directory structure (viz., Packaging a python library)project configuration pushed to setup.cfg (i.e., trivial setup.py and no requirements.txt)An example console scriptpytest configuration and example test under tests (i.e., outside of src)towncrier biolerplate for development / release note generationMinimal Dockerfile for containerized development and deploymentSphinx documentation boilerplate and a Makefile to automatically generate Sphinx API documentationA bunch of other boilerplate configuration files includingtox.ini supporting multiple Python version development and testing.editorconfig exported from Pycharm settings.gitignore generated by gitignore.ioNow What?I'll be dogfooding this, but I would love feedbackif anyone else decides to give it a spin. Drop me a comment on the blog or the project.Github pull requests welcome. Finalized at 12:45 PM. Tagged with programming and Python. Click to read and post comments Collecting Images for Classifers Dr. Bryan Patrick Wood June 05, 2021 Filed under “DS/AI/ML” Looks like I might beat my previous time between posts in a landslide. I was told it was about a year in between myfirst and second posts. Not fair as this one will be less content-rich. Also, please forgive the self-promotion.BackgroundI've always loved the quoteI’m a great believer inluck. The harder I work, the more luck I have.You're asked to build a model. Models need data. If you're lucky that data already exists somewhere or existing modelswhere trained with them in mind. Most of the time that's not the case.One class classificationis an entire topic itself, and my current thinking is typical may not be the best approach. Let's say it's abinary image classifier. Those typically need images of both positive and negative examples. Usually a lot of botheven when using transfer learning. That sounds like a huge pain. And it is.@bpw1621/imgscrapeThrew together something pretty quick to address a need. Done in some spare time over a weekend which makes thisa fairly rare instance of something shareable that was work-adjacent. Rough around the edges for sure but did the job itneeded to.More info about usage here.@bpw1621/imgscrape12 is a pretty simple node based image webscraper. It uses puppeteer for controlling thebrowser and provides a yargs based CLI interface. First npm module I have taken the time to publish (and glad tohave gone through that process now). Please visit the links for more information.For those that just want to use this as a tool, because it wasn't clear to meimmediately how to just install this and do that, it's as simple as, for instance, the followingnpx imgscrape-cli -t narwhal -e googleSome engines work better than others at the moment and all worked better when I hadfirst written it. I find Yandex usually works the best in terms of volume, usually in the thousands, while the rest stopin the hundreds of images. YMMV.The CodeAlmost all the logic is in lib/scrapeImages.js which clocks in at a little over 200 lines of code and should be prettyapproachable. The puppeteer package does all the heavy lifting here. Itsnode code so a lot of async and await which I prefer to callbacks and explicitly using promises given the choice.After instantiating the browser object, and a little more setup you're brought to a large switch statement with thedetails about the individual image search engines (e.g., URL, CSS selectors for the images, etc.). That part coulddefinitely use some refactoring. Next we go to the page and scroll down looking for images making sure to find thesite specific more results button if it pops up.Supports both URL and data images. There is also logic to try to determine if the engine is just returningduplicate images or has run out of results and bail if that is the case. This is another part that could use a look:it worked well when it was first written, but I think some engines changed aspects of their page results since then, andthose do not work great. Lastly, information about the successful, failed, and duplicate URLs are dumped out to JSONfiles along with the images.Yargs be a node.js library fer hearties tryin' ter parse optstrings. Love the whimsy ...The cli/imgscrape-cli.js parses setups up the CLI interface, parses the command lineoptions, and calls the scrapeImages function lib/scrapeImages using the yargs package. I had notused yargs before and ended up pleased with it. It supported subcommands, detailed options specifications, example ofcommands, aliases for long and short style options, and a couple other niceties. The API supports method chaining whichI also liked.Github Source Code ↩NPM package ↩ Finalized at 9:48 PM. Tagged with data-science, programming, Node, and Javascript. Click to read and post comments Retreat into the past→ Retreat into the past→ ©2020–2021 Dr. Bryan Patrick Wood. All rights reserved.
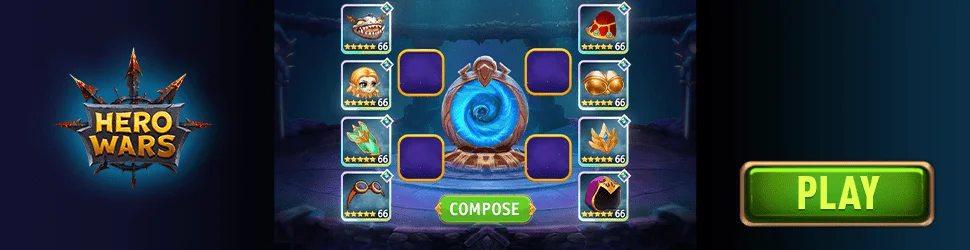
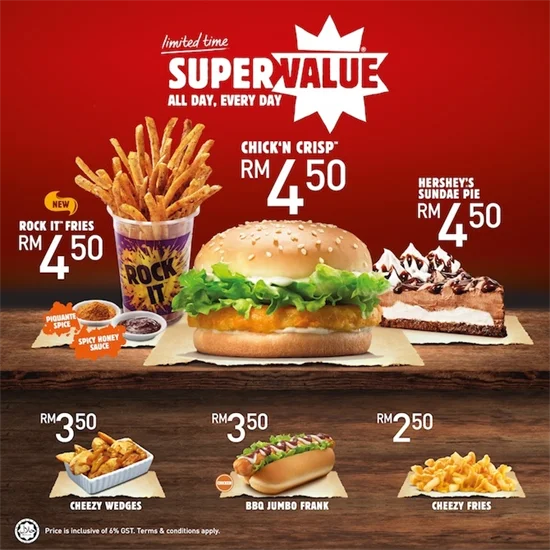
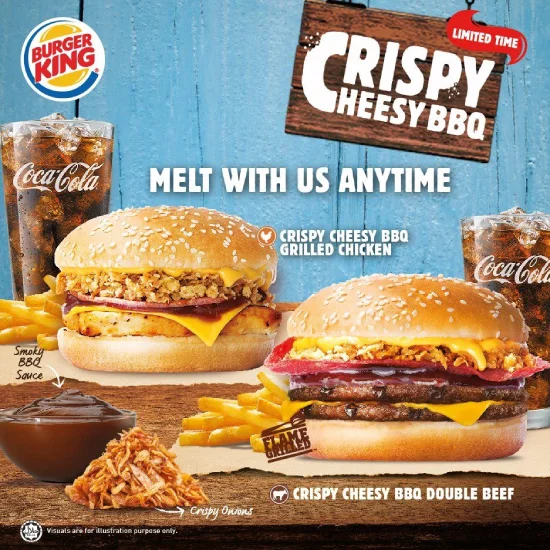