
"I love Sanjiv Kumar - Google Research"
www.sanjivk.com VS www.gqak.com
2022-07-06 07:56:39
Sanjiv Kumar PhD (2005; Robotics, SCS, CMU)Distinguished Research ScientistGoogle Research, NY76, Ninth AveNew York, NY 10011, USAemail: sanjivk AT google.comResearch InterestsLarge Scale Machine Learning, Artificial Intelligence, Health AI, Computer Vision, RoboticsTeachingEECS6898: Large-Scale Machine Learning, Fall 2010, Columbia University, New York, NY.TutorialsApproximate Nearest Neighbor Search (Trees and Hashes): Part-I, Part-II.Fast Matrix Decomposition: Part-I, Part-II. RecentPublications [ All Publications ] .A. K. Menon, A. S. Rawat, S. J. Reddi, S. Kim, and S. KumarA Statistical Perspective on DistillationInternational Conference on Machine Learning (ICML), 2021.[pdf] .A. S. Rawat, A. K. Menon, W. Jitkrittum, S. Jayasumana, F. X. Yu, S. J. Reddi, and S. KumarDisentangling Labeling and Sampling Bias for Learning in Large-output SpacesInternational Conference on Machine Learning (ICML), 2021.[pdf] .S. J. Reddi, R. K. Pasumarthi, A. K. Menon, A. S. Rawat, F. Yu, S. Kim, A. Veit, and S. KumarRankDistil: Knowledge Distillation for RankingInternational Conference on Artificial Intelligence and Statistics (AISTATS) 2021.[pdf] .A. K. Menon, A. S. Rawat, and S. KumarOverparameterisation and Worst-case Generalisation: Friend or Foe?International Conference on Learning Representations (ICLR), 2021.[pdf] .S. J. Reddi, Z. Charles, M. Zaheer, Z. Garrett, K. Rush, J. Konecn�, S. Kumar, and H. B. McMahan Adaptive Federated OptimizationInternational Conference on Learning Representations (ICLR), 2021.[pdf] .A. K. Menon, S. Jayasumana, A. S. Rawat, H. JainA. Veit and S. KumarLong-tail Learning via Logit AdjustmentInternational Conference on Learning Representations (ICLR), 2021.[pdf] .C.-Y. Hsieh, C.-K. Yeh, X. Liu, P. Ravikumar,S. Kim, S. Kumar, and C.-J. HsiehEvaluations and Methods for ExplanationThrough Robustness AnalysisInternational Conference on Learning Representations (ICLR), 2021.[pdf] .J. Zhang, A. K. Menon, A. Veit, S. Bhojanapalli, S. Kumar, and S. SraCoping With Label Shift via DistributionallyRobust OptimisationInternational Conference on Learning Representations (ICLR), 2021.[pdf] .C. Yun, Y.-W. Chang, S. Bhojanapalli, A. S. Rawat, S. Reddi, and S. KumarO(n) Connections are Expressive Enough:Universal Approximability of Sparse TransformersNeural Information Processing Systems (NeurIPS), 2020.[pdf] .J. Zhang, S. P. Karimireddy, A. Veit, S. Kim, S. Reddi, and S. KumarWhy are Adaptive Methods Goodfor Attention Models?Neural Information Processing Systems (NeurIPS), 2020.[pdf] .M. Weber, M. Zaheer, A. S. Rawat, A. Menon, and S. KumarRobust Large-Margin Learning in Hyperbolic SpaceNeural Information Processing Systems (NeurIPS), 2020.[pdf] .Y. Liu, A. T. Suresh, F. Yu, S. Kumar, and M. Riley Learning Discrete Distributions: User vs Item-LevelPrivacyNeural Information Processing Systems (NeurIPS), 2020.[pdf] .H. Chen, S. Si, Y. Li, C. Chelba, S. Kumar, D. Boning, and C.-J. Hsieh Multi-Stage Influence FunctionNeural Information Processing Systems (NeurIPS), 2020.[pdf] .S. Bhojanapalli, C. Yun, A. S. Rawat, S. Reddi, and S. Kumar Low-Rank Bottleneck in Multi-head Attention ModelsInternational Conference on Machine Learning (ICML), 2020.[pdf] .M. Lukasik, S. Bhojanapalli, A. K. Menon, and S. Kumar Does Label Smoothing Mitigate Label Noise?International Conference on Machine Learning (ICML), 2020.[pdf] .R. Guo, P. Sun, E. Lindgren, Q. Geng, D. Simcha, F. Chern and S. Kumar Accelerating Large-Scale Inference with Anisotropic Vector QuantizationInternational Conference on Machine Learning (ICML), 2020.[pdf] .F. X. Yu, A. S. Rawat, A. K. Menon, and S. Kumar Federated Learning with Only Positive LabelsInternational Conference on Machine Learning (ICML), 2020.[pdf] .Y. You, J. Li, S. Reddi, J. Hseu, S. Kumar, S. Bhojanapalli, X. Song, J. Demmel, K. Keutzer, and C.-J. Hsieh Large Batch Optimization for Deep Learning:Training BERT in 76 MinutesInternational Conference on Learning Representations (ICLR), 2020.[pdf] .C. Yun, S. Bhojanapalli, A. S. Rawat, S. J. Reddi, and S. Kumar Are Transformers Universal Approximatorsof Sequence-to-Sequence Functions?International Conference on Learning Representations (ICLR), 2020.[pdf] .A. K. Menon, A. S. Rawat, S. J. Reddi, and S. Kumar Can Gradient Clipping Mitigate Label Noise?International Conference on Learning Representations (ICLR), 2020.[pdf] .W.-C. Chang, F. Yu, Y.-W. Chang, and S. Kumar Pre-training Tasks for Embedding-based Large-scale RetrievalInternational Conference on Learning Representations (ICLR), 2020.[pdf] .Y. Ruan, Y. Xiong, S. Reddi, S. Kumar,C.-J. Hsieh Learning to Learn by Zeroth-Order OracleInternational Conference on Learning Representations (ICLR), 2020.[pdf] .C.-J. Hsieh, Q. Cao, S. Kumar, S. Si, T. Xiao, and X. Liu How Does Noise Help Robustness? Explanation and Exploration under the Neural SDE FrameworkInternational Conference on Computer Vision and Pattern Recognition (CVPR), 2020.[pdf] .A. K. Menon, A. S. Rawat, S. J. Reddi, and S. Kumar Multilabel Reductions: What is My Loss Optimising?Neural Information Processing Systems (NeurIPS), 2019.[pdf] .C. Guo, A. Mousavi, X. Wu, D. Holtmann-Rice, S. Kale, S. Reddi and S. KumarBreaking the Glass Ceiling for Embedding-BasedClassifiers for Large Output SpacesNeural Information Processing Systems (NeurIPS), 2019.[pdf] .A. S. Rawat, J. Chen, F. Yu, A. T. Suresh, and S. KumarSampled Softmax with Random Fourier FeaturesNeural Information Processing Systems (NeurIPS), 2019.[pdf] .M. Staib, S. Reddi, S. Kale, S. Kumar, and S. Sra Escaping Saddle Points with Adaptive Gradient MethodsInternational Conference on Machine Learning (ICML), 2019.[pdf] .S. Wu, A. G. Dimakis, S. Sanghavi, F. X. Yu, D. Holtmann-Rice, D. Storcheus, A. Rostamizadeh, and S. KumarLearning a Compressed Sensing Measurement Matrix via Gradient UnrollingInternational Conference on Machine Learning (ICML), 2019.[pdf] .P.-H. (Patrick) Chen, S. Si, S. Kumar, Y. Li, and C.-J. HsiehLearning to Screen for Fast Softmax Inference on Large Vocabulary Neural NetworksInternational Conference on Learning Representations (ICLR), 2019.[pdf] .S. Reddi, S. Kale, F. X. Yu, D. Holtmann-Rice, J. chen and S. KumarStochastic Negative Mining for Learning with Large Output SpacesInternational Conference on Artificial Intelligence and Statistics (AISTATS), 2019.[pdf] .Q. Geng, W. Ding, R. Guo, and S. KumarOptimal Noise-Adding Mechanism in Additive Differential PrivacyInternational Conference on Artificial Intelligence and Statistics (AISTATS), 2019.[pdf] .S. Reddi, M. Zaheer, D. Sachan, S. Kale, and S. KumarAdaptive Methods for Nonconvex OptimizationNeural Information Processing Systems (NIPS), 2018.[pdf] .N. Agarwal, A. T. Suresh, F. X. Yu, S. Kumar, and H. B. McMahancpSGD: Communication-efficient anddifferentially-private distributed SGDNeural Information Processing Systems (NIPS), 2018.[pdf] .Ian E. H. Yen, S. Kale, F. X. Yu, D. Holtmann-Rice, S. Kumar, P. RavikumarLoss Decomposition for Fast Learning in Large Output SpacesInternational Conference on Machine Learning (ICML), 2018.[pdf] .S. Reddi, S. Kale, S. Kumar [best paper award] On the Convergence of Adam and Beyond International Conference on Learning Representations (ICLR), 2018.[pdf] .S. Si, S. Kumar, Y. LiNonlinear Online Learning with Adaptive Nystrom ApproximationarXiv:1802.07887, 2018.[pdf] .X. Wu, R. Guo, A. T. Suresh, S. Kumar, D. Holtmann-Rice, D. Simcha, F. X. YuMultiscale Quantization for Fast Similarity SearchNeural Information Processing Systems (NIPS), 2017.[pdf] .B. Dai, R. Guo, S. Kumar, N. He, L. SongStochastic Generative HashingInternational Conference on Machine Learning (ICML), 2017.[pdf] .A. T. Suresh, F. X. Yu, S. Kumar, H. B. McMahanDistributed Mean Estimation with Limited CommunicationInternational Conference on Machine Learning (ICML), 2017.[pdf] .X. Zhang, F. X. Yu, S. Kumar, S. F. ChangLearning Spread-out Local Feature DescriptorsInternational Conference on Computer Vision (ICCV), 2017.[pdf] .K. Zhong, R. Guo, S. Kumar, B. Yan, D. Simcha, I. S. DhillonFast Classification with Binary PrototypesInternational Conference on Artificial Intelligence and Statistics (AISTATS), 2017.[pdf] .F. X. Yu, A. T. Suresh, K. Choromanski, D. Holtmann-Rice, S. KumarOrthogonal Random FeaturesNeural Information Processing Systems (NIPS), 2016.[pdf] .A. Choromanska, K. Choromanski, M. Bojarski, T. Jebara, S. Kumar, Y. LeCunBinary Embeddings with Structured Hash ProjectionsInternational Conference on Machine Learning (ICML), 2016.[pdf] .R. Guo, S. Kumar, K. Choromanski, and D. SimchaQuantization based Fast Inner Product SearchInternational Conference on Artificial Intelligence and Statistics (AISTATS), 2016. [pdf]Previous Arxiv version: arXiv:1509.01469, 2015. [pdf].J. Wang, W. Liu, S. Kumar, S. F. ChangLearning to Hash for Indexing Big Data - A SurveyProceedings of the IEEE, Volume: 104, Issue: 1 , Jan. 2016.[pdf] .J. Pennington, F. X. Yu, S. KumarSpherical Random Features for Polynomial KernelsNeural Information Processing Systems (NIPS), 2015.[pdf] .V. Sindhwani, T. Sainath, S. KumarStructured Transforms for Small-Footprint Deep LearningNeural Information Processing Systems (NIPS), 2015.[pdf] .X. Zhang, F. X. Yu, Ruiqi Guo, S. Kumar, S. Wang, S.-F. ChangFast Orthogonal Projection Based on Kronecker ProductInternational Conference on Computer Vision (ICCV), 2015.[pdf] .Y. Cheng, F. X. Yu, R. S. Feris, S. Kumar,A. Choudhary, and S. F. ChangAn Exploration of Parameter Redundancy in Deep Networks withCirculant ProjectionsInternational Conference on Computer Vision (ICCV), 2015.[pdf]Previous arXiv version: arXiv:1502.03436v1, 2015. [pdf].K. Choromanski, S. Kumar, and X. LiuFast Online Clustering with Randomized Skeleton SetsarXiv:1506.03425v1, 2015.[pdf].F. X. Yu, S. Kumar,H. Rowley, and S. F. ChangCompact Nonlinear Maps and Circulant ExtensionsarXiv:1503.03893v1, 2015.[pdf].F. X. Yu, Y. Gong, and S. Kumar Fast Binary Embedding for High-Dimensional DataBook Chapter, Multimedia Data Mining and Analytics, 2015.[pdf].W. Liu, C. Mu, S. Kumar,and S. F. ChangDiscrete Graph HashingNeural Information Processing Systems (NIPS), 2014.[pdf]Supplementary material can be found here..F. X. Yu, S. Kumar, Y. Gong,and S. F. ChangCirculant Binary EmbeddingInternational Conference on Machine Learning (ICML), 2014.[pdf]Matlab code can be found here..F. X. Yu, D. Liu, S. Kumar, T. Jebara,and S. F. ChangpSVM for Learning with Label ProportionsInternational Conference on Machine Learning (ICML), 2013.[pdf]The supplementary file with additional proofs and experiments is here..Y. Gong, S. Kumar, H. Rowley,and S. LazebnikLearning Binary Codes for High-Dimensional Data Using Bilinear ProjectionsIEEE Computer Vision and Pattern Recognition (CVPR), 2013.[pdf].A. Talwalkar, S. Kumar, M. Mohriand H. RowleyLarge-scale SVD and Manifold LearningJournal of Machine Learning Research (JMLR), 2013.[pdf].Y. Gong, S. Kumar, V. Verma,and S. LazebnikAngular Quantization-based Binary Codes for Fast Similarity SearchAdvances in Neural Information Processing Systems (NIPS), 2012.[pdf].J. He, S. Kumar,and S. F. ChangOn the Difficulty of Nearest Neighbor SearchInternational Conference on Machine Learning (ICML), 2012.[pdf]The supplementary file containing all the proofs is here.NOTE: This is a slightly edited version of what is in the ICML proceedings..W. Liu, J. Wang, Y. Mu, S. Kumar,and S. F. ChangCompact Hyperplane Hashing with Bilinear FunctionsInternational Conference on Machine Learning (ICML), 2012.[pdf]The supplementary file containing extended proofs and results is here..J. Wang, S. Kumar,and S. F. ChangSemi-Supervised Hashing for Large Scale SearchIEEE Transactions on Pattern Analysis and Machine Intelligence (PAMI), 2012.[pdf].S. Kumar, M. Mohriand A. TalwalkarSampling Methods for the Nystrom MethodJournal of Machine Learning Research (JMLR), 2012.[pdf].W. Liu, J. Wang, S. Kumar,and S. F. ChangHashing with Graphs International Conference onMachine Learning (ICML), 2011.[pdf].A. Talwalkar, S. Kumar, M. Mohriand H. RowleyLarge-Scale Manifold LearningBook chapter in Manifold Learning Theory and Applications. Editors: Y. Ma and Y. Fu. CRC Press, 2011.[pdf].S. Kumar,M. Mohri and A. TalwalkarEnsemble NystromBook chapter in Ensemble Machine Learning: Theory and Applications, Springer, 2011.[pdf]Modified to correct an error in the computational complexity analysis. April 2011..A. Makadia, V. Pavlovic and S. KumarBaselines for Image AnnotationInternational Journal on Computer Vision (IJCV), 2010.[pdf].J. Wang, S. Kumar,and S. F. ChangSequential Projection Learning for Hashing with Compact Codes International Conference onMachine Learning (ICML), 2010.[pdf].Z. Wang, M. Zhao, Y. Song, S. Kumar and B. LiYouTubeCat: Learning to Categorize Wild Web VideosIEEE Computer Vision and Pattern Recognition (CVPR), 2010.[pdf].J. Wang, S. Kumar,and S. F. ChangSemi-Supervised Hashing for Scalable Image RetrievalIEEE Computer Vision and Pattern Recognition (CVPR), 2010.[pdf]Typo in Eq (21) corrected. June 2010..S. Kumar Discriminative Graphical Models for Context-Based ClassificationBook chapter in Computer Vision: Detection, Recognition and Reconstruction, Springer, 2010.Eds. R. Cipolla, S. Battiato, and G. M. Farinella.[pdf].S. Kumar,M. Mohri and A. TalwalkarEnsemble Nystrom Method Neural Information Processing Systems(NIPS), 2009.[pdf]Modified to correct an error in the computational complexity analysis. April 2011..S. Kumar,M. Mohri and A. TalwalkarOn Sampling-based Approximate Spectral Decomposition International Conference onMachine Learning (ICML), 2009.[pdf].S. Kumar,M. Mohri and A. TalwalkarSampling Techniques for the Nystrom Method Twelfth International Conference onArtificial Intelligence and Statistics (AISTATS), 2009.[pdf].A. Makadia,V. Pavlovic and S. Kumar A New Baseline for Image AnnotationEuropean Conference on Computer Vision (ECCV), 2008.[pdf].A. Talwalkar, S. Kumar andH. A. RowleyLarge-Scale Manifold LearningIEEE Computer Vision and Pattern Recognition (CVPR), 2008.[pdf].M. Kim,S. Kumar, V. Pavlovic andH. A. RowleyFace Tracking and Recognition withVisual Constraints in Real-World VideosIEEE Computer Vision and Pattern Recognition (CVPR), 2008.[pdf].S. Kumar andH. A. RowleyClassification of Weakly-Labeled Datawith Partial Equivalence RelationsIEEE International Conference on ComputerVision (ICCV), 2007.[pdf]Some additional results and parts of thevideo and retrieval datasets used in this work can be seen here.�S. Kumar and M. HebertDiscriminative Random FieldsInternational Journal of Computer Vision(IJCV), 68(2), 179-201, 2006.[pdf] �S. Kumar,J. August and M. HebertExploiting Inference for ApproximateParameter Learning in Discriminative Fields: An Empirical StudyEnergy Minimization Methods in Computer Vision and Pattern Recognition(EMMCVPR), 2005.[pdf] This paper is an extended and revised version of the earlierwork presented in Snowbird Learning Workshop, 2004.�S. KumarModels for Learning Spatial Interactionsin Natural Images for Context-Based ClassificationPhD Thesis, The Robotics Institute, School of Computer Science,Carnegie Mellon University, September 2005.[pdf] [ps]Revised October 2005.�S.Kumar and M. HebertA Hierarchical Field Framework for UnifiedContext-Based ClassificationIEEE International Conference on ComputerVision (ICCV), 2005.[pdf] [ps]Revised October 2005.�C. Rother, S. Kumar, V.Kolmogorov and A. BlakeDigital TapestryIEEE International Conference on Computer Vision andPattern Recognition (CVPR), June, 2005.[pdf]�S. Kumar and M. HebertApproximate Parameter Learning in DiscriminativeFieldsSnowbird Learning Workshop, Utah, 2004.[pdf] [ps]The synthetic dataset used for learning and inferenceexperiments can be obtained from here.�S. Kumar and M. HebertMulticlass Discriminative Fields for Parts-BasedObject DetectionSnowbird Learning Workshop, Utah, 2004.[pdf]�S. Kumar and M. HebertDiscriminative Fields for Modeling SpatialDependencies in Natural ImagesAdvances in NeuralInformation Processing Systems, NIPS 16, 2004.[pdf] [ps]The binary denoising synthetic dataset used fortraining and testing can be obtained from here.�B. Nabbe, S. Kumar, andM. Hebert Path Planningwith Hallucinated Worlds InProc. IEEE/RSJ International Conference on Intelligent Robots and Systems(IROS), October 2004. [pdf] �S.Kumar and M. HebertDiscriminative Random Fields: A DiscriminativeFramework for Contextual Interaction in ClassificationIEEE International Conference on Computer Vision(ICCV), 2003.[pdf] [ps]�S. Kumar and M. HebertMan-Made Structure Detection in Natural Images usinga Causal Multiscale Random FieldIEEE International Conference on Computer Vision andPattern Recognition (CVPR), 2003.[pdf]Some more example resultsand comparisons.The structure detection database used for trainingand testing can be obtained from here.�S. Kumar, A. C. Loui,and M. HebertAn Observation-Constrained Generative Approach forProbabilistic Classification of Image Regions Image and Vision Computing, 21,pp. 87-97, 2003.[pdf] A shorter version of this paperappeared in the following workshop:�S. Kumar, A. C. Loui,and M. HebertProbabilistic Classification of Image Regions usingan Observation-Constrained Generative ApproachECCV Workshop on Generative Modelsbased Vision (GMBV), 2002. [pdf]
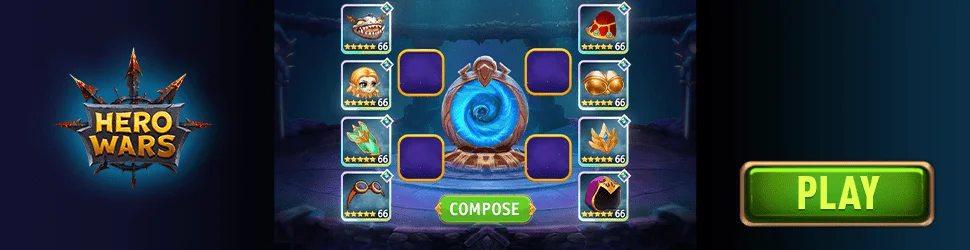
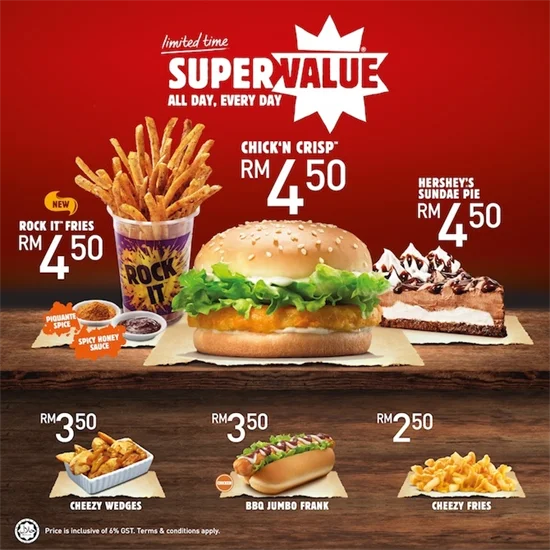
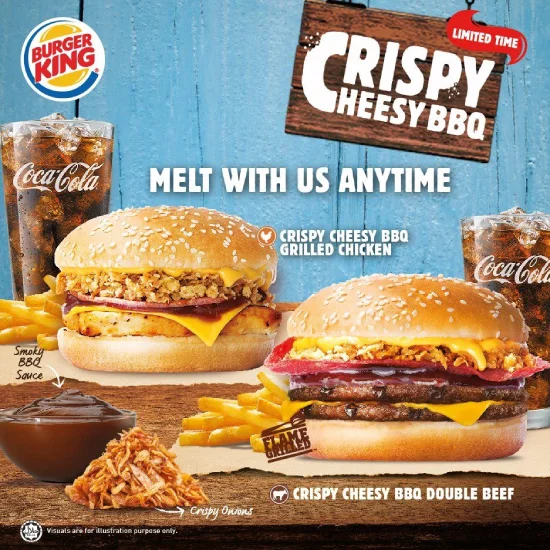