
"I love Trochaisms | Letters make words; sentences make paragraphs"
www.trochee.net VS www.gqak.com
2022-08-08 05:16:18
TrochaismsLetters make words; sentences make paragraphs Skip to contentHomeAboutWorkCromulatronSamyro← Older postsGreater data science, part 1.1: the undisciplinedPosted on June 21, 2016 by JeremyThis is part of an open-ended series of marginalia to Denoho’s 50 Years of Data Science 2015 paper.Fields that aren’t disciplinesAs discussed previously, disciplines are fields that have all three of :content: the field has something to say,organizational structure: the field has well-formed ways of saying it, anda standard of validity: a way in which the field admits new contributions.Tukey & Donoho’s three categories make a nice Venn diagram (pace Drew Conway). Continue reading →Posted in data science, Patterns, work|1 CommentApropos of software engineering for scientists, I had the opportunity to be a reviewer for C. Titus Brown‘s JOSS publication of sourmash, is a pretty cool Python library around some very fast C code for computing (and comparing) MinHash sketches on (gene) sequences.My critique of sourmash is marked “minor revisions only” because the core functionality is so useful and usefully abstracted (while staying close to the usual formats in genetics). There are lots of nice things about the way Titus has packaged it, and I threw a few more suggestions into the github issue tracker. I enjoyed getting to look through this package, and I want to promote sourmash as a model for how scientific packages should be shared within and across labs, at least in Python.Posted on June 17, 2016 by Jeremy|1 CommentGreater data science, part 2.1 – software engineering for scientistsPosted on June 16, 2016 by JeremyThis is part of an open-ended series of marginalia to Donoho’s 50 Years of Data Science 2015 paper.In many scientific labs, the skills and knowledge required for the research (e.g. linguistics fieldwork, sociological interview practices, wet-lab biological analysis) are not the same skills involved in software engineering or in data curation and maintenance.Some scientists thus find themselves as the “accidental techie” in their local lab — maybe not even as the “accidental data scientist“, but doing specific software engineering tasks — you’re the poor schmuck stuck making sure that everybody’s spreadsheets validate, that nobody sorts the data on the wrong field, that you removed the “track changes” history the proposal before you sent it off to the grant agencies, etc.Scientific labs of any scale (including academic labs, though they probably don’t have the budgets or the incentives) can really benefit from data science, but especially software engineering expertise, even — or perhaps especially — when the engineer isn’t an inside-baseball expert in the research domain. I list below a number of places an experienced software engineer (or data scientist) can make a difference to a field she (or he) doesn’t know well. Continue reading →Posted in data science, programming, statistics, work|6 CommentsI’ve written a half dozen pieces of commentary on David Donoho’s work, all the while spelling his name wrong; at least once in a permalink URL. Oh, well. At least I can edit the posts here.Posted on June 16, 2016 by Jeremy|1 CommentGreater data science, part 2: data science for scientistsPosted on June 16, 2016 by JeremyThis is part of an open-ended series of marginalia to Donoho’s 50 Years of Data Science 2015 paper.Many aspects of Donoho’s 2015 “greater data science” can support scientists of other stripes — and not just because “data scientist is like food cook” — if data science is a thing after all, then it has specific expertise that applies to shared problems across domains. I have been thinking a lot about how the outsider-ish nature of the “data science” can provide supporting analysis in a specific domain-tied (“wet-lab”) science.This is not to dismiss the data science that’s already happening in the wet-lab — but to acknowledge that the expertise of the data scientist is often complementary to the domain expertise of her wet-lab colleague.Here I lay out three classes of skills that I’ve seen in “data scientists” (more rarely, but still sometimes, in software engineers, or in target-domain experts: these people might be called the “accidental data scientists”, if it’s not circular).“Direct” data scienceDonoho 2015 includes six divisions of “greater data science”:Greater Data Science is all opportunities to help out “other” sciences.methodological review on data collection and transformationrepresentational review ensuring that — where possible — the best standards for data representation are available; this is a sort of future-proofing and also feeds into cross-methodological analyses (below)statistical methods review on core and peripheral models and analysesvisualization and presentation design and review, to support exploration of input data and post-analysis datacross-methodological analyses are much easier to adapt when data representations and transformations conform to agreed-upon standardsCoping with “big” dataadaptation of methods for large-scale data cross-cuts most of the above — understanding how to adapt analytic methods to “embarrassingly parallel” architecturesrefusing to adapt methods for large-scale data when, for example, the data really aren’t as large as all that. Remember, many analyses can be run on a single machine with a few thousand dollars’ worth of RAM and disk, rather than requiring a compute cluster at orders of magnitude more expense. (Of course, projects like Apache Beam aim to bake in the ability to scale down, but this is by no means mature.)pipeline audit capacity — visualization and other insight into data at intermediate stages of processing is more important the larger the scale of the dataScientific honesty and client relationshipsdata scientists are in a uniquely well-suited position to actually improve the human quality of the “wet lab” research scientists they support. By focusing on the data science in particular, they can:identify publication bias, or other temptations like p-hacking, even if inadvertent (these may also be part of the statistical methods review above)support good-faith re-analysis when mistakes are discovered in the upstream data, the pipelines or supporting packages: if you’re doing all the software work above, re-running should be easyact as a “subjects’ ombuds[wo]man” by considering (e.g.) the privacy and reward trade-offs in the analytics workflow and the risks of data leakagefacilitate the communication within and between labsfind ways to automate the boring and mechanical parts of the data pipeline processPosted in data science, programming, statistics|3 CommentsGreater data science, part 1: the disciplinePosted on June 13, 2016 by JeremyThis is part of an open-ended series of marginalia to Donoho’s 50 Years of Data Science 2015 paper.Donoho compares “data science” (or “data analysis”, a term he inherits from John Tukey) to statistics in terms of three foundational conditions, quoting Tukey:These three are the answers to “what”, “how”, and “why” — for any science.Let’s call these three core conditions content, structure, and (a means of determining) validity. Anything with an answer in all three rows we might call a discipline, but we distinguish among disciplines by their choice of validity conditions.Disciplines without empiricismTukey (and Donoho) suggest that a science is determined by using experience (or predictive power) as their means of validity. Tukey himself declared mathematics a different kind of discipline thus:By these tests mathematics is not a science, since its ultimate standard of validity is an agreed-upon sort of logical consistency and provability.Other disciplines may use different validity conditions:Mathematics uses a “sort of… provability” as its standard of validity, as claimed by Tukey himself.New Criticism and American Protestantism both make claims based on the literality (the “immediateness” of their respective reference texts) to uphold their validity.Some branches of linguistics and philosophy appeal to explanatory simplicity as a standard of validity (but I see you, Minimalism — you just pushed the complexity somewhere else and declared it not interesting; that’s called “ignoring externalities”).Secular judicial law, the Talmud, the Hadiths, Roman Catholic papal decrees, and the Marvel No-Prize all incorporate adherence to precedent as a standard of validity.I don’t mean to dismiss fields that use something other than “experience” (empiricism) as a standard of validity (though that standard is obviously appealing). Other standards are relevant even within fields we might still consider sciences: the Bohr model of the atom is a form that can be explained to high school students, and it has validity because it’s an effective scaffold for teaching, not because it’s the most accurate in experiment (it’s known to be wrong).Posted in data science, math, Patterns, programming, statistics|1 CommentI was going to post a follow up entry or two on data science, but then a red-blooded American homophobic terrorist went and murdered fifty people in Orlando with a military weapon, because they were GLBTQ, or because they were Latinx, or both.And another one got stopped on his way to Pride in LA, or he might have succeeded in doing the same.Love your fellow humans. Love their love; love that they love; love that they love who they love.Be humane. Be humble. Be human. Stop the killing.Posted on June 12, 2016 by Jeremy|1 CommentSome wise thoughts from my complementary-distribution doppelganger Bill McNeill, currently occupying our ecological niche in Austin:IDE-independence has a lot of advantages.IDEs are CodeSmellPosted on June 10, 2016 by Jeremy|3 CommentsHey, nifty. I just found out that you can write RMarkdown-style literate Python files and use the Jupyter notebook environment to view and execute them (with the notedown package, which also allows you to edit them in place). This has nice implications for source control — changes to ipython notebooks are pretty ugly.Posted on June 8, 2016 by Jeremy|1 CommentDonoho’s “Greater Data Science’, part 0Posted on June 7, 2016 by Jeremy“50 years of Data Science”. Donoho, David. 2015. [link to downloadable versions]Donoho’s got a manifesto that ain’t foolin’ around. I have a lot of thoughts about it, but I’m going to write them up as an open-ended series of marginalia on this remarkable essay.Data science is a thing after allI’ve said elsewhere (probably also elsewhere on this blog) that I’m not sure “data science” is a thing: to paraphrase Dorothy Parker Aldous Huxley, data science has always seemed like “72 nine suburbs in search of a city metropolis”.But I’m here to bring the good word: Greater Data Science, as Denoho describes it, probably is a thing. Continue reading →Posted in academics, data science, programming, statistics|4 Comments← Older postsRecent PostsGreater data science, part 1.1: the undisciplinedreviewing software engineering for scientists – sourmashGreater data science, part 2.1 – software engineering for scientistsspelling be hardGreater data science, part 2: data science for scientistsRecent Commentstrochee on Greater data science, part 1.1: the undisciplinedtrochee on reviewing software engineering for scientists – sourmashBill McNeill on Greater data science, part 2.1 – software engineering for scientistsJeremy on Greater data science, part 2.1 – software engineering for scientistskylewadegrove on Greater data science, part 2: data science for scientistsArchivesJune 2016May 2016April 2016March 2016September 2013May 2013April 2013March 2013November 2012October 2012September 2012August 2012July 2012June 2012May 2012April 2012March 2012February 2012January 2012December 2011November 2011October 2011September 2011August 2011July 2011June 2011May 2011April 2011Categoriesacademicsadminasidedata sciencedoggerelinformation theorylinguisticsmathPatternsplaceholderpoliticsprogrammingsamyroSan Franciscoscience fictionSeattleSFstatisticstechTwitterlogUncategorizedworkMetaLog inEntries RSSComments RSSWordPress.orgTrochaismsProudly powered by WordPress.
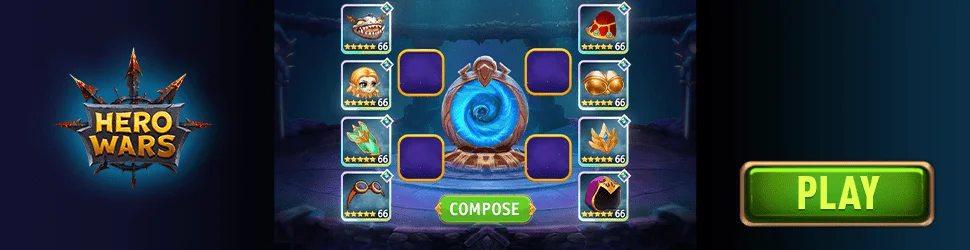
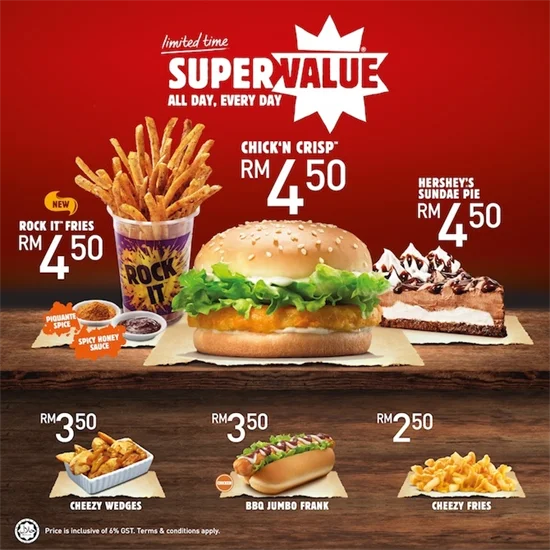
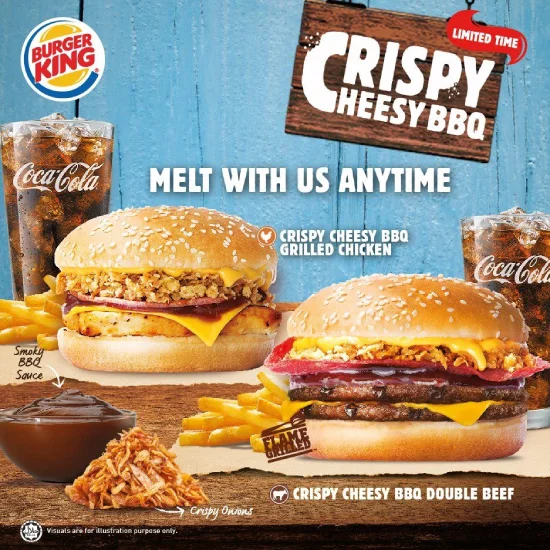